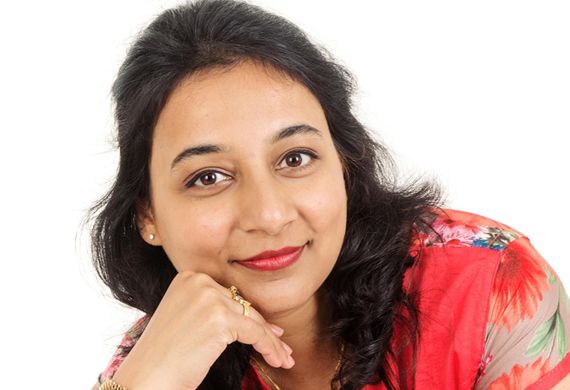
Blending Innovation & Accountability in Leading Model Risk Management
By: Nidhi Agarwal, Chief Model Risk Officer, Virgin Money
Nidhi Agarwal leads the development and maintenance of credit risk models at Virgin Money, overseeing AI/GenAI capabilities and model risk frameworks. Previously, she spent 12+ years at HSBC, most recently as Global Head of Model Risk Evaluation. She also runs a UK charity and is an award-winning author.
In an insightful interaction with Women Entrepreneurs Review Magazine, Nidhi shares her insights on managing the entire model lifecycle, balancing compliance with independence, and leveraging model risk management as a strategic advantage. She also reflected on key lessons and unconventional metrics for navigating economic volatility.
You're known for managing the entire model lifecycle, from development to validation. If your risk models had a personality, what would be their most distinguishing trait?
If risk models had a personality, they would be quick-witted and futuristic, using lessons from the past to make the right decisions for the future. Resilient in market shocks, they’d adapt by constantly analyzing patterns and staying alert to new data. They would evolve over time to account for changes in the environment.
The challenge is balancing their autonomy with the need for oversight. Regular validation, robust testing, and continuous monitoring help ensure models stay aligned with current conditions, while still allowing them to make independent decisions. This balance lets models learn and evolve, while safety guardrails are in place to prevent unexpected outcomes.
As a woman leader deeply involved in model governance, what’s your secret sauce for making sure models don’t get too big for their boots and stay compliant with regulations?
Model governance is all about balancing innovation with accountability. My "secret sauce" involves three key ingredients: ensuring there is proactive monitoring, model outcomes can be explained and that there is cross-functional collaboration between the three lines of defence to ensure compliance at any point of time.
Sometimes models do act funnily - but on deeper analysis it is always because some underlying assumption made by the model has changed. When we were looking to grow our credit cards portfolio by making the product proposition more attractive, the model initially misbehaved and gave better outputs than it should have. The commercial teams celebrated the high predicted returns until we quickly recalibrated the model to the higher risk levels we were seeing in applications.
In the world of complex credit and market risk models, how do you make sure your team stays motivated, especially when a model is giving you the silent treatment during its validation phase?
Our work is critical—for the bank but also for customers who trust us with their hard-earned money. This sense of purpose keeps the team motivated, especially during challenging times. When a model refuses to cooperate, we remind ourselves the stakes are high: a misaligned model can mean incorrect risk assessments, poor business decisions, or regulatory non-compliance—consequences too significant to ignore.
Our pep talks always bring us back to this bigger picture. Regular model monitoring detects deviations between expected and actual outcomes, allowing us to recalibrate and refine. When the model finally falls in line after tweaks, there’s always a collective sigh of relief—and a good laugh. It’s like convincing a stubborn child to finish their homework; sometimes, you just have to appreciate the effort!
In navigating volatile economic conditions, how can model risk management serve as a strategic advantage? What unconventional metrics should leaders is looking at?
Proactively managing model risk can ensure models remain resilient, adaptable, and capable of making sound predictions even in an unstable environment. The key is viewing model risk management as a dynamic process, not a one-and-done, meaningit becomes a tool to build trust with stakeholders, manage unforeseen risks, and maintain strategic agility.
Some unconventional metrics to look at: Robustness under Stress - evaluating how the model performs under stress scenarios, Model Drift - monitoring for shifts in predictions over time by spotting gradual misalignments rather than immediate errors, Data Dependency - monitoring variables the model is most reliant on and tracking their stability, Model Agility – time needed to recalibrate following significant shifts in market or credit conditions. If a model is too slow to adjust, it might be a risk rather than a strategic advantage.
If you could go back in time to when you first started in risk management, what advice would you give your younger self about managing risk in models?
Understand the Model’s Purpose and Limitations – No model is perfect. Every model makes assumptions, and those assumptions introduce risk. Always question what a model is meant to do and where it might fail. Models are powerful, but they are just tools, not infallible solutions. It’s important to be open to constant feedback, validation, and iteration. Managing model risk is as much about fostering a culture of continuous learning and adaptation as it is about creating complex algorithms.
Models may speak in numbers, but their implications often affect business decisions, so understanding how to translate model behavior into actionable insights is critical. It’s essential to clearly communicate the assumptions, limitations, and risks of a model to both technical and non-technical stakeholders.